Large Language Models (LLMs) are powerful Generative AI models that can learn statistical relationships between words, which enables them to generate human-like text, translate languages, write different kinds of creative content, and answer questions in an informative way. Since the birth of the Transformer architecture introduced in the “Attention Is All You Need” paper, we have seen the emergence of increasingly powerful LLMs.
However, Large Language Models (LLMs) by themselves are not enough. This is because of two key reasons. First, they tend to hallucinate, which means they can “make up” facts and information that are simply untrue. LLMs work by predicting the next token in a sequence and are inherently probabilistic. This means they can generate factually incorrect statements, especially when prompted on topics outside their training data or when the training data itself is inaccurate.
This brings us to the second limitation: companies looking to build AI applications using LLMs that leverage their internal data cannot solely rely on these models, as they are limited to the data on which they were originally trained.
To bypass the above limitations, the performance of an LLM can be augmented by connecting it to an external data source. Here’s how it works: upon receiving a query, relevant information is fetched from the data source and sent to the LLM before response generation. This way, the behavior of an LLM can be ‘grounded,’ while also harnessing its analytical capabilities. This approach is known as a Retrieval Augmented Generation (RAG) system.
Making Your Data AI-Ready
Effective AI requires the right data in the right place. Understanding data types is crucial to this process. Here’s what it takes to get data AI-ready and how to handle massive amounts of relevant data:
- Data Types: Identify and classify different data types your AI will use. Structured data (like databases) and unstructured data (like text and images) need different handling techniques.
- Data Preparation: Clean, normalize, and format your data to ensure it’s usable by AI systems. This involves removing duplicates, correcting errors, and standardizing formats.
- Storage Solutions: Opt for scalable storage solutions that can handle large volumes of data efficiently. Cloud-based storage options offer flexibility and scalability.
- Data Integration: Ensure seamless integration of data from multiple sources. This can involve using APIs, middleware, or data lakes to consolidate information.
- Ongoing Management: Regularly update and maintain your data to keep it relevant and accurate. Implement data governance policies to manage data quality and compliance.
This comprehensive approach ensures that your data is not just available but optimized for AI applications, enhancing both performance and reliability. Whether you’re just beginning your AI journey or are well underway, having AI-ready data is a key component to success.
One of the most powerful aspects of the RAG architecture is its ability to unlock the knowledge stored in unstructured data in addition to structured data. In fact, a significant portion of data globally—estimated at 80% to 90% by various analysts—is unstructured, and this is a huge untapped resource for companies to leverage. This makes LLM-powered RAG applications one of the most powerful approaches in the AI domain.
Knowledge Graphs and Vector Databases are two widely used technologies for building RAG applications. They differ significantly in terms of the underlying abstractions they use to operate and, as a result, offer different capabilities for data querying and extraction.
This blog will help you understand when and why to choose either of these technologies so that you can make the most of using AI to understand and leverage your data.
What is a Knowledge Graph?
A Knowledge Graph is a structured representation of information. It organizes data into nodes (entities) and edges (the relationships between them).
Here’s a simple example of a Knowledge Graph around the game of football.
- Lionel Messi “plays for” Paris Saint-Germain (PSG)
- Lionel Messi “represents” Argentina
- Cristiano Ronaldo “plays for” Manchester United
- Cristiano Ronaldo “represents” Portugal
- Paris Saint-Germain (PSG) “competes in” UEFA Champions League
- Manchester United “competes in” UEFA Champions League
- Argentina “competes in” FIFA World Cup
- Portugal “competes in” FIFA World Cup
- Mauricio Pochettino “manages” Paris Saint-Germain (PSG)
- Ole Gunnar Solskjær “manages” Manchester United
The words in bold represent the entities, and the ones in inverted commas are the relationships between these entities. It has been said that the human brain behaves like a Knowledge Graph and, therefore, this way of structuring data makes it highly human-readable.
Knowledge Graphs are very useful in discovering deep and intricate connections in your data. This enables complex querying capabilities. For example, you could ask:
“Find all players who have played under Pep Guardiola at both FC Barcelona and Manchester City, and have also scored in a UEFA Champions League final for either of these clubs.”
A Knowledge Graph will be able to handle this query by traversing the relationships between different entities such as players, managers, clubs, and match events.
There are several popular Knowledge Graphs that are publicly available:
- Wikidata
- Freebase
- YAGO
- DBpedia
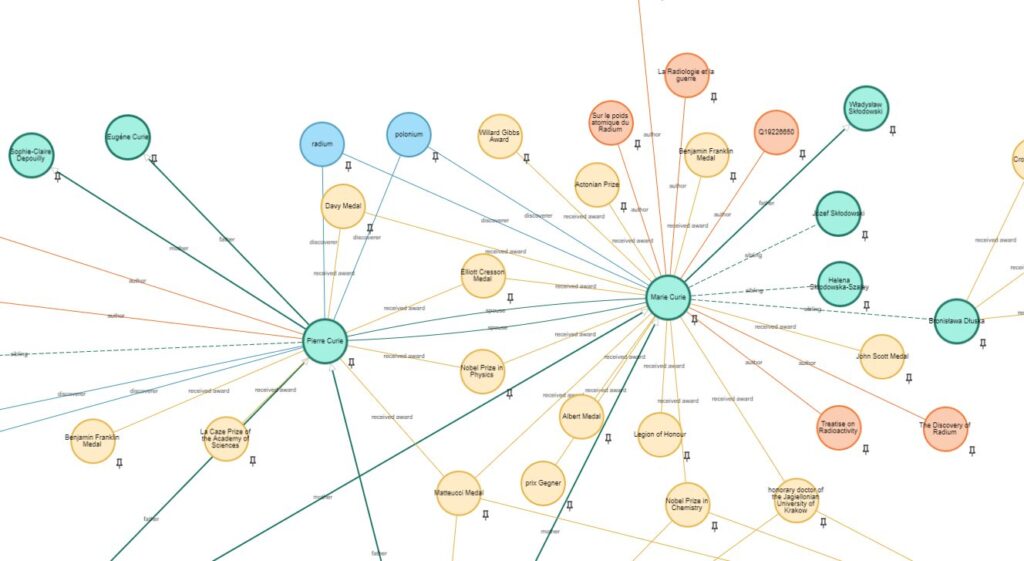
You are, however, not limited to using these available Knowledge Graphs to build your applications; any form of unstructured data can be modeled as a Knowledge Graph. For instance, consider a company with a repository of customer service emails. By extracting key entities and relationships from these emails, such as customer names, issues, resolutions, and timestamps, you can create a Knowledge Graph that maps the interactions and solutions. Similarly, you can identify relationships between objects in an image and model these connections as a Knowledge Graph to build image clustering and recognition algorithms.
Knowledge Graphs are stored in specialized databases that are designed to handle Cypher, a powerful query language specifically designed for interacting with graph databases. The Knowledge Graph Database, also known as Graph Database, is optimized for traversing and manipulating graph structures, and is the fundamental building block behind Knowledge Graph-powered RAG applications (GraphRAG).
Understanding Depth Parameters in Graph Databases
Depth parameters play a crucial role in navigating and analyzing relationships within graph databases. They help define the extent of traversal allowed from a starting node to target nodes, making it easier to uncover complex relationships.
What Are Depth Parameters?
Depth parameters determine the minimum and maximum number of hops (or relationships) traversed from one node to another. These parameters are highly useful in identifying various levels of connections within a dataset. They allow for more controlled and precise queries, ensuring that only relevant data is retrieved without overwhelming the system with unnecessary information.
Implementing Depth Parameters in Cypher Queries
In Cypher, a query language for graph databases, depth parameters are specified directly in the relationship patterns. Here’s a step-by-step breakdown of how to incorporate them:
Define the Relationship Ranges:
Specify the range of relationships (hops) between nodes. This can be done by using an asterisk (*) followed by the range. For instance,*1..20
indicates a minimum of 1 hop and a maximum of 20 hops.Incorporate Depth in Relationships:
Use the depth parameters within the relationship definitions in your Cypher query. This is done by specifying the range within square brackets.Formulate the Complete Query:
Construct a query to navigate from a starting node to target nodes, using the defined depth parameters to control the traversal.
Here’s an example query that demonstrates these steps:
MATCH path = (exec:EXECUTIVE)-[:REPORTS*1..20]->(metric:FINANCIAL_METRIC)-[:IMPACTED_BY*1..20]->(cond:MARKET_CONDITION)
WHERE exec.name = 'Tim Cook'
RETURN exec, metric, cond, path;
In this query:
[:REPORTS*1..20] specifies that the traversal from the EXECUTIVE node to the FINANCIAL_METRIC node should involve a minimum of 1 and a maximum of 20 relationships.
Similarly, [:IMPACTED_BY*1..20] indicates the depth for traversing from FINANCIAL_METRIC to MARKET_CONDITION.
Benefits of Using Depth Parameters
- Enhanced Precision: Return only the most relevant data subsets by limiting the depth of traversal.
- Performance Optimization: Improve query efficiency by avoiding unnecessary deep traversals.
- Flexibility: Easily adjustable ranges to fit varying requirements of relationship analysis.
In summary, depth parameters are essential tools in Cypher queries for managing the complexity of relationships in graph databases. They provide both precision and flexibility, ensuring efficient and targeted data retrieval.
What is a Vector Database?
A Vector Database stores unstructured data like text, audio, and images in the form of numerical vectors, also known as embeddings. This allows for efficient similarity searches as data points with semantic relatedness are closer to each other in the vector space.
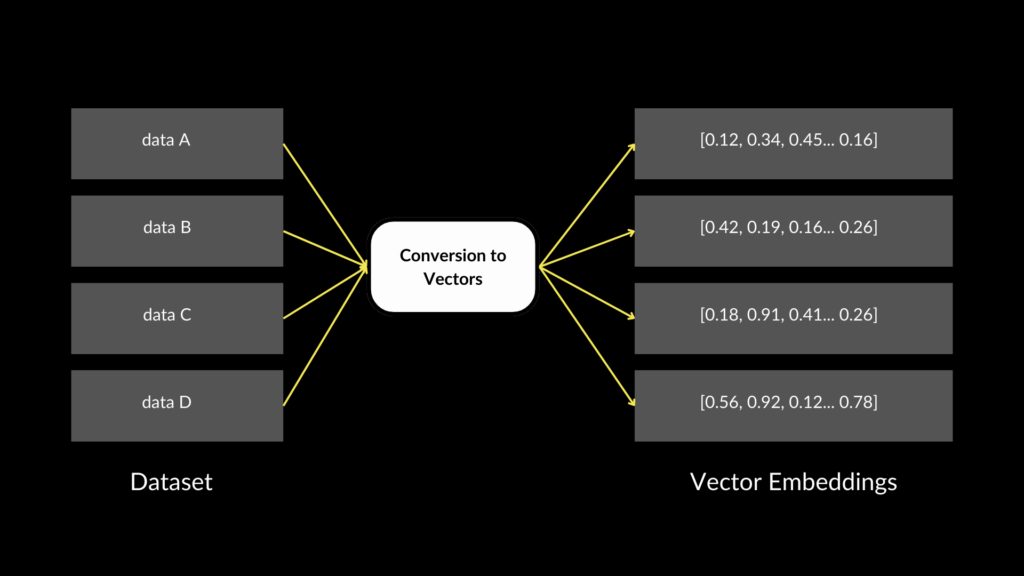
Vector Databases are designed to optimize and search through massive datasets to find points that are semantically similar to the query. For example, they are good at handling queries like:
- “Find news articles related to the recent transfer of Cristiano Ronaldo”
- “Retrieve Match Reports on Recent High-Scoring Games”
- “Discover opinion pieces on Emerging Football Talents”
- “Locate Player Profiles similar to Rising Stars”
However, since Vector Databases store information as discrete vectors representing individual text chunks, they miss out on the intricate relationships and contextual connections between these chunks. A similarity search can often be too broad and, therefore, not suitable for precise question-answering. For instance, while a Vector Database can efficiently find articles related to a specific player’s transfer, it might struggle with more intricate queries that require a deeper understanding of relational data, such as:
“Identify all players who have been transferred from a club in the English Premier League to a club in La Liga, and have won a league title in both leagues.”
Vector databases excel at identifying semantically similar objects, but they fall short in capturing the underlying knowledge that Knowledge Graphs do so well.
Knowledge Graphs: Pros and Cons
Pros
- Complex Question-Answering
Knowledge Graphs excel at answering complex queries that involve hopping through several entities and relationships. They can efficiently navigate graph structures to provide precise answers. This makes them ideal for applications that require detailed insights or multi-step reasoning.
Using graph data and adding edges gives AI developers control over query results, improving relevancy and increasing the reliability of their AI applications. By altering relationship models within these graphs, developers can fine-tune the connections between entities to reflect more accurate and contextually relevant information.
Key Benefits for AI Developers:
- Improved Relevancy: Adjusting the edges and relationships directly impacts the accuracy of query results, ensuring that the information retrieved is pertinent and useful.
- Increased Reliability: Fine-tuning the graph structure enhances the overall reliability of the AI application, making it more dependable in various scenarios.
- Flexibility: The ability to modify relationship models allows for continuous optimization and adaptation to new data or changing requirements.
By leveraging these capabilities, AI developers can create more sophisticated and reliable applications that meet the demands of complex, data-driven environments.
Enhancing vector queries by including graph data significantly improves query relevance. Benchmarks show query accuracy improving 2.8x with the addition of knowledge to complex vector queries. This combination leverages the strengths of both vector and graph data, providing a robust solution for tackling intricate queries.
Incorporating graph data into your query framework not only boosts accuracy but also enhances the depth of the insights generated. By enabling multi-hop reasoning and detailed entity relationships, knowledge graphs transform the way complex queries are handled, offering unparalleled precision and efficiency.
- Credible Response
The structured nature of Knowledge Graphs allows for reliable and accurate responses. For example, when querying for “all Nobel Prize winners in Physics,” a Knowledge Graph can provide a precise list because it stores verified relationships and entity attributes.
- Human-Readable
Knowledge Graphs are designed to be easily interpretable by humans, and you can create visualizations from them. By being able to see the relationships and hierarchies at a glance, you can assess whether the Knowledge Graph captures the nuances needed and identify areas for improvement in data modeling.
- Transparency and Explainability
The transparency of Knowledge Graphs is particularly valuable when dealing with errors or inaccuracies, and helps in building Explainable AI applications. If a KG-RAG application makes an error, you can easily trace it back to the specific node or relationship in the Knowledge Graph where the incorrect information originated from and correct it.
Cons
- Requires Data Modeling
Building a Knowledge Graph requires the data to be modeled into entity-relationship-entity triples. This can be a complex process and requires a deep understanding of the domain. To simplify this process, there are emerging techniques such as REBEL or using pre-trained LLMs.
- Missing Out on Broad Results
While Knowledge Graphs are excellent for specific, complex queries, they may miss out on broader results that don’t fit neatly into the predefined schema.
Vector Databases: Pros and Cons
Pros
- Handling Unstructured Data
Vector databases are adept at handling a wide range of unstructured data types. By converting this data into high-dimensional vectors, these databases can efficiently store and retrieve information that doesn’t fit neatly into traditional schemas.
- Effective Retrieval of Documents
Vector Databases excel at retrieving documents based on semantic similarity, which means they can fetch information even when exact keywords aren’t present. This makes them highly effective for tasks like searching through a large corpus of articles – for example, legal documents containing specialized language and terminology about specific laws.
Cons
- Struggling with Complex Relationships
Since Vector Databases solely rely on finding similarities in data, they struggle with queries that involve figuring out complex relationships between entities.
- Can Generate Incorrect Information
Reliance on semantic similarity can often lead to answers that are factually incorrect. For example, the query “Find doctors who specialize in cardiology” might return results that include nurses or technicians who have worked in cardiology departments, but are not doctors. In order to fix this, developers often need to employ additional techniques, such as reranking.
- Impossible to Achieve Exact Results
Vector Databases have predefined limits in responses, which can result in either incomplete or overly broad results. For example, for the query “Employees who joined the company in 2021”, the vector search could return an incomplete list of employees if the set limit is too low. Conversely, if the limit is set higher, the results could also include employees who did not join in 2021 because of the semantic metric. Developers struggle to tweak these predefined limits to achieve exact results, as there is no clear basis for adjusting them.
- Lacks Data Transparency
Vector Databases are essentially black boxes since data is transformed into numerical vectors. Therefore, if there is an error in the response of an LLM, it can be rather difficult to trace back to the source of the error. The opaque nature of vector representations obscures the path from input to output. This makes it challenging to build Explainable AI systems with Vector Databases.
Knowledge Graphs vs Vector Databases: Key Differences
Where KGs Win
Since knowledge graphs knowledge graphs try to capture the intrinsic knowledge hidden within data, it is easier to build reliable and accurate RAG applications using knowledge graphs rather than with vector databases. Additionally, knowledge graphs can be more efficient due to their structured and compressed nature. Vector databases often require significant memory overhead due to high-dimensional vector storage and indexing needs.
Vector databases sometimes require additional reranking AI modules to refine results due to predefined limits on initial data retrieval accuracy. This creates more complexity in the application architecture. knowledge graphs are often more self-complete and provide refined results without such additional steps.
Graph structures help create levers for breadth and depth for answer retrieval. Using a real-world financial analysis example, we saw that graph structures give far more leverage for creating more complete answers in both depth and breadth. They also create a semantically consistent, accurate, and deterministic way to perform information retrieval.
Using graph structures in conjunction with vector search promises a high level of deterministic and complete retrieval, which is crucial for enterprise workflows. By combining the strengths of both technologies, organizations can ensure high accuracy and comprehensive results, enhancing the efficiency and reliability of their data retrieval systems.
Where Vector Databases Win
Unstructured Data can be easily converted to vector embeddings and imported in bulk into Vector Databases. In contrast, data intended for Knowledge Graphs require structuring, including the definition of entities and relationships, as well as the generation of Cypher queries or similar query languages.
The table below highlights some of the key differences between Vector Databases and Knowledge Graphs:
Parameter | Knowledge Graph | Vector Database |
---|---|---|
Data Representation | Graph structure with nodes (entities) and edges (relationships) | High-dimensional vectors representing data points |
Purpose | To model relationships and interconnections between entities | To store and retrieve data based on similarity in vector space |
Data Type | Structured and semi-structured data | Unstructured data such as text, images, and multimedia |
Use Cases | Complex Querying and Relationship Analysis, Data Management & Organization | Semantic Search, Document Retrieval |
Scalability | Scales with complexity of relationships and entities | Scales with the number of vectors and dimensions |
Data Updates | Can automatically update relationships with new data | Requires retraining or reindexing vectors for updates |
Performance | Efficient for relationship-based queries | Efficient for similarity-based queries |
Data Storage | Typically uses RDF, property graphs | Uses vector storage formats optimized for similarity search |
Example Technologies | FalkorDB, Neo4j, NebulaGraph | Pinecone, Qdrant, Weaviate |
Similarities Between Knowledge Graphs and Vector Databases
As we have seen, the two are quite different from each other. Despite that, they are quite similar in their application use cases. Here are some similarities:
Purpose
Both are used to store and query relationships between data points. This helps in effectively augmenting an LLM’s response by quickly finding data relevant to the query.
Scalability
Both scale with data complexity, whether relationships (Knowledge Graphs) or volume and dimensionality (Vector Databases).
Integration
Both can integrate with machine learning (ML) and AI technologies.
Use Cases
Both are used in recommendation systems, fraud detection, AI-powered searches, and data discovery.
Real-Time Analytics
Both are indexed and optimized for fast data insertion and retrieval. This makes them ideal for applications that require real-time updates.
When to Use a Knowledge Graph?
- Structured Data and Relationships: Use KGs when complex relationships between structured data entities need to be managed. Knowledge Graphs perform excellently in scenarios where the interconnections between data points are essential, increasing search engine capabilities. For example, enterprise data management platforms.
- Domain-Specific Applications: KGs can be particularly useful for applications requiring deep, domain-specific knowledge. For example, they can be specialized in effectively providing answers in domains such as healthcare and clinical decision support or Search Engine Optimization (SEO).
- Explainability and Traceability: KGs offer more transparent reasoning paths if the application requires a high degree of explainability or reasoning (i.e., understanding how a conclusion was reached). For example, in financial services and fraud detection, where institutions need to explain why certain transactions are flagged as suspicious.
- Data Integrity and Consistency: KGs maintain data integrity and are suitable when consistency in data representation is crucial. For example, LinkedIn’s Knowledge Graph reflects consistent changes across all platforms, which is how it provides accurate job recommendations and networking opportunities.
When to Use a Vector Database?
- Unstructured Data: Vector databases are adept at capturing the semantic meaning of large volumes of unstructured data, such as text, images, or audio. For example, Pinterest can retrieve similar images by comparing images based on visual features, enabling advanced search and recommendation functionalities for users.
- Broad Retrieval: Vector Databases are more suitable for applications that require broad retrieval with vague querying. For example, semantic searching can quickly dig out relevant information in a job search engine to display resumes based on limited job descriptions.
- Flexibility in Data Modeling: A Vector Database can be more appropriate if there is a need to incorporate diverse data types quickly. For example, in the domain of real-time social media monitoring, organizations can quickly analyze vast amounts of text, videos, and audio streams without having to worry about modeling the schemas beforehand.
Are Knowledge Graphs Better Than Vector Databases for LLM Hallucinations?
As discussed earlier, Knowledge Graphs are much better at generating factually correct and precise responses. This is because verified knowledge can be accessed and retrieved by traversing through nodes and relationships.
Vector Databases, on the other hand, are designed to handle massive unstructured data. However, the similarity search metric can introduce noise in the information, resulting in less precise answers.
Combining Knowledge Graphs and Vector Databases
Both Knowledge Graphs and Vector Databases are powerful knowledge representation techniques, each with its own strengths and weaknesses. Vector Databases are particularly effective at determining similarity between concepts, but they struggle with evaluating complex dependencies and other logic-based operations, which are the strengths of Knowledge Graphs.
By combining these two approaches, you can create a unified system that leverages the complementary strengths of both. It can help you achieve broad semantic similarity as well as robust logical reasoning in your NLP and AI applications. This dual approach, therefore, provides a more comprehensive understanding of data and can be a powerful tactic for powering more advanced use cases of RAG applications.
How Knowledge Graphs Enhance Vector Search Results
Knowledge graphs provide a way of dramatically improving query results because they give you the ability to add and remove links between related contexts LLMs need. This dynamic management of links enhances the flexibility and adaptiveness of the search process, allowing for more precise and relevant query results.
Here’s how it can work: start with an initial vector search, where a user query is transformed into a high-dimensional vector representation. This vector is then used to perform a similarity search within the vector index, and it retrieves the top K most semantically similar nodes. This is then used as the initial context for the Knowledge Graph traversal. This helps reach important fragments of data scattered across the graph, creating a richer and more comprehensive context than using vector search or graph traversal alone.
Using graph data and adding edges gives AI developers control over query results, improving relevancy and increasing the reliability of their AI applications. By incorporating Vector Graph, developers can easily alter relationship models, enhancing the relevancy of queries. This simple, drop-in enhancement has consistently improved the performance of AI queries compared to plain vector queries. However, it is worth noting that creating graph relationships can be resource-intensive.
In essence, the combination of vector searches and Knowledge Graph traversal not only captures semantically similar nodes but also enriches the context by understanding the relationships between data points. This dual approach ensures that AI applications deliver more accurate and reliable results, making it a powerful tool for developers aiming to optimize query relevance.But how exactly do we combine depth and breadth searches to retrieve these complex sub-graphs with rich insights?
Detailed Use Case
Imagine we want to analyze how a global corporation’s strategic decisions impact its financial metrics across different geographic segments over several quarters. For instance, understanding how executive decisions influence product development and market conditions in regions like the Americas, Europe and Japan. We could start by structuring a graph query that represents these interconnected relationships among various entities:
MATCH (exec:EXECUTIVE)-[r1:REPORTS]->(metric:FINANCIAL_METRIC),
(metric)-[r2:IMPACTED_BY]->(cond:MARKET_CONDITION),
(prod:PRODUCT)-[r3:PRESENTS]->(metric),
(prod)-[r4:OPERATES_IN]->(geo:GEOGRAPHIC_SEGMENT),
(event:EVENT)-[r5:OCCURS_DURING]->(time:TIME_PERIOD),
(event)-[r6:IMPACTS]->(metric)
WHERE exec.name IN ['Alice Smith', 'John Doe'] AND
geo.name IN ['Americas', 'Europe', 'Japan'] AND
time.name IN ['Q1 2023', 'Q2 2023', 'Q3 2023']
RETURN exec, metric, cond, prod, geo, event, time, r1,r2,r3,r4,r5,r6
This query enables us to visualize and analyze intricate relationships between executives, financial metrics, products, geographic segments, market conditions, and time periods.
Combining Vector Search and Graph Traversal
The initial vector search provides a broad overview by identifying semantically similar nodes, which serves as the starting point. Next, the graph traversal dives deeper, exploring the specific relationships and nodes defined in the query. By leveraging both methods, we can uncover nuanced insights that might be missed if only one method were used.
Contextual Retrieval
Furthermore, using this combined approach allows for enhanced contextual retrieval. The initial vector search sets the stage, while the graph traversal fills in the details, ensuring that no critical piece of information is overlooked. This dual method creates a comprehensive and multifaceted view of the data, providing richer insights and more informed decision-making.
By structuring our approach this way, we maximize the strengths of both vector search and graph traversal, effectively combining depth and breadth searches to retrieve complex sub-graphs with rich insights.
Challenges in Integrating Knowledge Graphs with Vector Databases
As we discussed above, combining these two technologies makes sense based on everything mentioned, especially for specific, complex tasks. However, most systems currently are being created as independent silos, meaning that data in Knowledge Graphs and Vector Databases are not inherently designed to work together.
This presents several challenges when building AI applications:
- Integration Challenges: Building applications where data resides in both databases is inherently difficult. Any updates to data would need to be synced across both systems, which further complicates development and maintenance.
- Query Latency: Combining data from these two separate databases adds significant overhead during the query retrieval step, and can introduce latency.
- Scaling Complexity: Each database type has different scaling characteristics and requirements. Therefore, it can become a challenge for developers or DevOps teams to manage both in production environments.
The right approach is an integrated system that leverages the powerful capabilities of Knowledge Graphs while also incorporating the semantic similarity capabilities of vector indexes.
How FalkorDB Can Help
FalkorDB provides a unified solution that seamlessly integrates the capabilities of Knowledge Graphs and Vector Databases. Its low-latency, Redis-powered architecture is designed to efficiently handle both graph traversal and vector similarity searches, thus eliminating the need for separate systems and reducing integration complexity.
Key Features of FalkorDB:
- Unified Data Storage: FalkorDB allows the storage of vector indexes alongside Knowledge Graph entities, and enables efficient querying of both graph and semantic data within a single database.
- Optimized Query Techniques: FalkorDB offers advanced query optimization techniques to ensure the efficient execution of complex queries that span both vector and graph data.
- Scalable Performance: FalkorDB’s architecture is designed for high performance and scalability. The system ensures fast response times even as data volumes grow.
- Simplified Maintenance: By integrating Knowledge Graph and Vector Database functionalities, FalkorDB reduces the operational complexity of maintaining separate systems.
How to Get Data AI-Ready: Necessary Steps Explained
Preparing data for AI involves several important steps to ensure accuracy and efficiency. Here’s a breakdown of what’s required:
1. Identify and Collect Relevant Data
- Determine Data Sources: Identify which datasets are relevant to your specific AI application.
- Gather Data: Utilize APIs, web scraping tools, or direct data imports to collect the necessary information.
2. Data Cleaning and Preprocessing
- Handle Missing Data: Impute missing values or remove incomplete records to maintain data integrity.
- Remove Duplicates: Ensure the dataset is free of duplicate entries that can skew results.
- Standardize Formats: Convert all data into a uniform format for consistency.
3. Data Transformation
- Normalization and Scaling: Adjust values to a common scale, especially important in algorithms that rely on distance measures.
- Encoding Categorical Variables: Convert categorical data into numerical values using techniques like one-hot encoding.
4. Split Data into Training and Testing Sets
- Create Training Set: Use a majority of the data (typically 70-80%) for training your AI model.
- Create Testing Set: Reserve the remaining data to validate and test the model’s accuracy.
5. Feature Selection and Engineering
- Select Key Features: Identify the most critical variables that will impact model performance.
- Engineer New Features: Create new variables that can provide more insights and improve the model’s predictive power.
6. Ensure Data Quality and Security
- Quality Checks: Conduct periodic audits to check for and rectify data quality issues.
- Data Security: Implement encryption and secure access protocols to protect sensitive information.
7. Data Integration and Storage
- Integrate Various Data Sources: Combine data from different sources to build a comprehensive dataset.
- Choose the Right Storage Solutions: Consider scalable storage solutions like cloud services to handle extensive datasets effectively.
Why This Matters
Understanding and executing these steps is key for optimal AI performance. No matter where you are on your AI development, having AI-ready data ensures that your models are accurate, reliable, and scalable.
By following these steps, you set a strong foundation that supports the development of robust AI systems capable of making meaningful predictions and decisions.
FalkorDB already works seamlessly with technologies like LangChain, Diffbot API, OpenAI, and LLMs like the Llama or Mistral series. For instance, when developing a RAG application, you can use FalkorDB to construct a Knowledge Graph from diverse documents, which can then serve as a rich contextual base for LLMs to generate more informed and precise answers. This approach not only enhances the capabilities of LLMs but also mitigates common issues like hallucinations by providing a solid factual foundation from the Knowledge Graphs.
Here’s how to get started:
- Visit the FalkorDB Website: Head over to FalkorDB’s official website to learn more about its features, capabilities, and how it can benefit your AI projects.
- Try FalkorDB: Follow the instructions on the website to download FalkorDB and install it using Docker for an easy setup process. Alternatively, start with FalkorDB Cloud for free.
- Explore the Documentation: Check out the comprehensive documentation available to understand how to effectively integrate FalkorDB into your existing systems.
- Join the Community: Engage with the FalkorDB community by joining our Discord channel, reading blog posts, and participating in discussions to gain insights, share experiences, and stay updated on the latest developments.
Contact Support: If you have any questions or need assistance, don’t hesitate to reach out to our team.