Highlights
- KPMG AI Report cites data quality (85%) and privacy (71%) as top enterprise AI concerns; GraphRAG addresses both through structured retrieval.
- Enterprise trust in AI requires explainable outputs; GraphRAG uniquely provides transparent reasoning beyond basic embedding models.
- GraphRAG ensures tenant isolation, enabling secure AI agent implementations without risking organizational data leakage.
Enterprises are pushing AI agents from experimental stages into full production under intense pressures to deliver measurable value.
Yet, a key hurdle remains: traditional database architectures. These architectures obscure critical data relationships, undermining AI accuracy, privacy, and scalability. The KPMG AI Report confirms this, identifying data quality as the dominant challenge faced by 85% of enterprise leaders.
Vector databases—tabular schemas and document stores—seem logical but secretly mask complex data interdependencies. These structures fail to clearly represent the intricate relationships within modern, complex datasets.
As AI scales, hidden inconsistencies in traditional databases begin to surface, resulting in unreliable AI performance and unmanageable complexity.
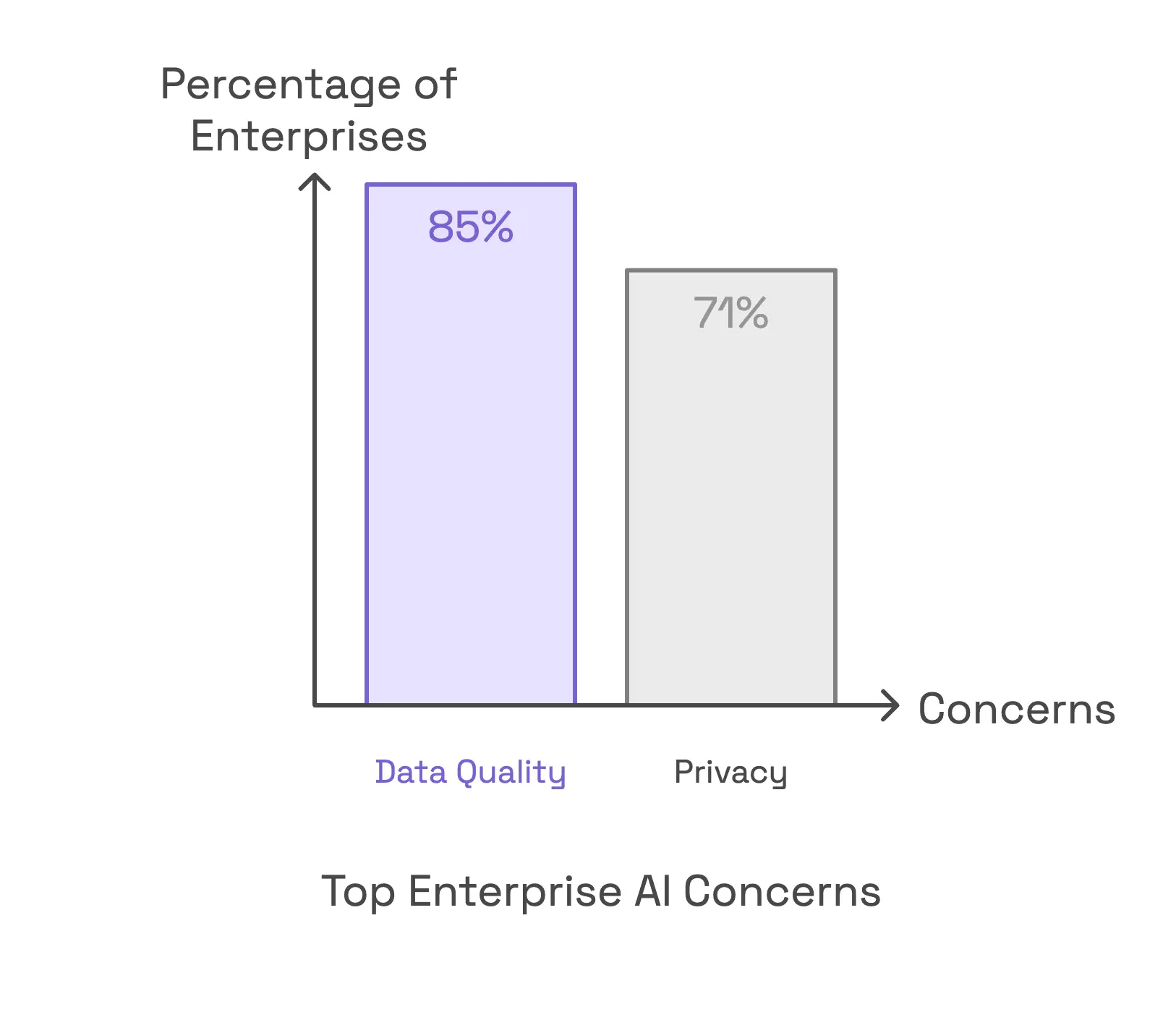
Scaling AI from Pilot to Production
Despite significant experimentation, 51% of organizations exploring AI agents and 37% piloting—only 12% achieve full operational deployment. Traditional database architectures struggle with real-time integration, flexible scalability, and operational integration, creating barriers to demonstrating ROI.
How GraphRAG Reframes the Data Quality Challenge
GraphRAG provides a new perspective by explicitly mapping data relationships, directly addressing the structural limitations inherent in conventional databases. This explicit representation dramatically enhances data clarity and integrity, which is foundational for reliable AI outcomes. By eliminating hidden inconsistencies, GraphRAG ensures enterprises can confidently scale their AI initiatives.
Correcting Misconceptions Directly
Misconception: Incremental database improvements suffice.
Misconception: External governance sufficiently protects data privacy.
Misconception: Embedding-based AI decisions offer adequate explainability.
Building Trust Through Explainability
AI adoption often stalls due to stakeholders’ skepticism about AI decision-making processes. Transparency and explainability are not just desirable—they’re essential. GraphRAG addresses this critical demand by providing explicit, traceable data retrieval pathways. Unlike traditional AI systems that rely on opaque embeddings, GraphRAG enables clear, defensible AI decisions, significantly boosting stakeholder confidence.
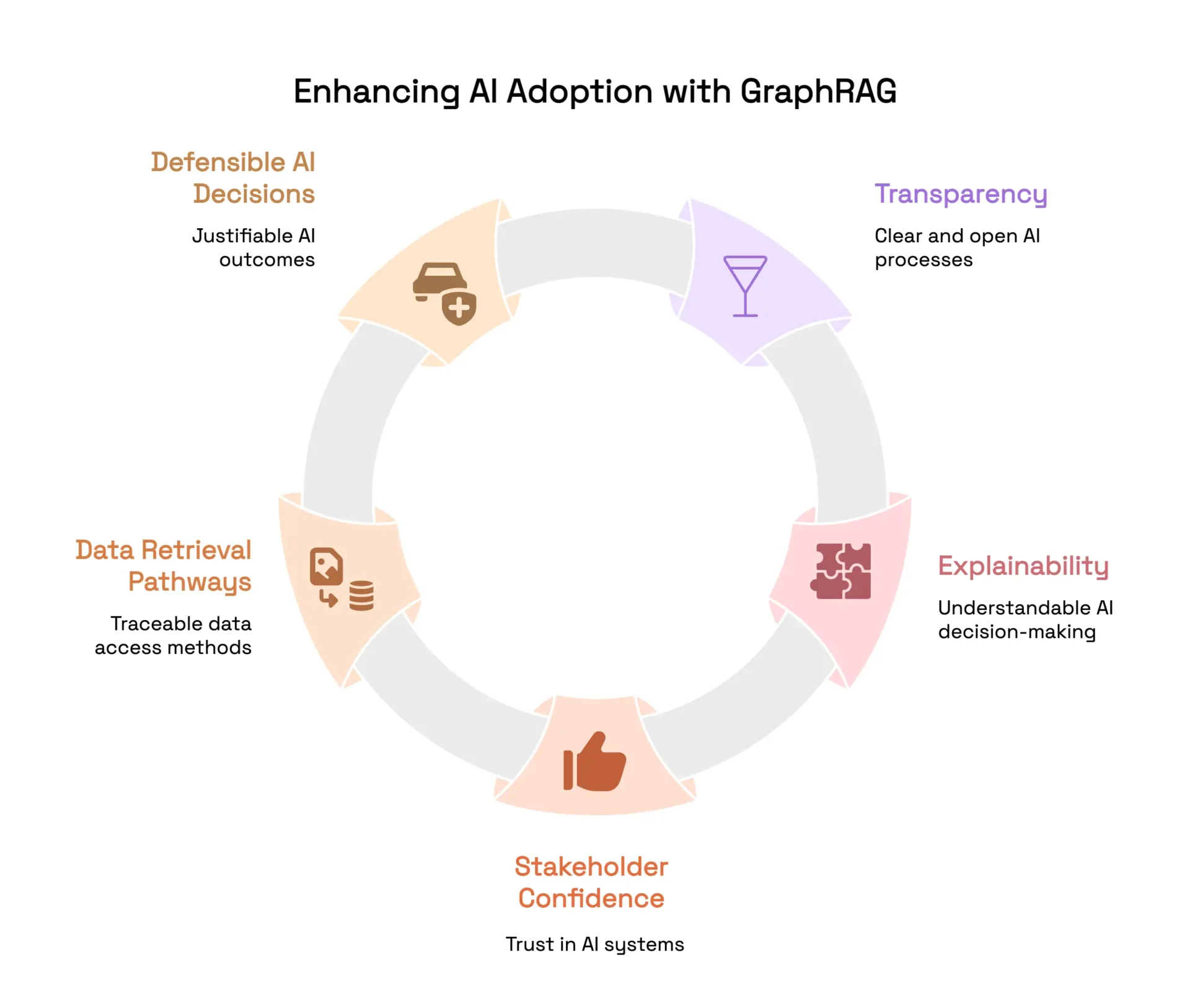
Privacy and cybersecurity remain top concerns for enterprises adopting AI, as highlighted by 71% of leaders in the KPMG AI Report. GraphRAG integrates security directly into its architecture through native tenant isolation. This design ensures data privacy and robust compliance, reducing reliance on external governance systems and minimizing vulnerabilities.
Enterprises continue to struggle with moving AI agents from pilot stages to fully operational deployments. The transition demands flexibility, seamless integration, and scalability—capabilities often missing in traditional databases. GraphRAG’s architecture inherently supports real-time data streams and horizontal scalability, simplifying the deployment process and bridging the critical gap from pilot to production.
If you’re still relying on traditional databases, you’re treating symptoms—not the root issue. Is your approach to AI adoption treating symptoms rather than underlying data complexity?
What key challenges does the KPMG AI Report highlight
Why is GraphRAG critical according to the KPMG AI Report findings?
How does GraphRAG differ from vector databases?
Build fast and accurate GenAI apps with GraphRAG SDK at scale
FalkorDB offers an accurate, multi-tenant RAG solution based on our low-latency, scalable graph database technology. It’s ideal for highly technical teams that handle complex, interconnected data in real-time, resulting in fewer hallucinations and more accurate responses from LLMs.